Forex forex mechanical systems trading
In September I published a post about the results of our random forest model for out of sample predictions in our price action based system repository at Asirikuy. The aim of this model is to use the in-sample results of trading strategies — meaning their back-testing results — to predict the first six months of real out-of-sample performance that is, the results of the first 6 months of live trading.
Forex Trading System
In this post I talked about prediction thresholds and how a 0. In order to address the above I repeated the Bayesian analysis I carried out last time in order to see how variables change as a function of the model classification threshold, whether the same threshold remains optimal and whether there is any change in how the posterior probability and performance values behave.
Forex Mechanical Trading Systems: What Every Trader Should Know
This is necessary because if we just perform random splits over the data we see artificially better results since we get data in the training set that could never be there given that the data is not generated all at the same time but across multiple market conditions that evolve as a function of time. The dataset is much larger with a total size of points compared to less than points when this analysis was last done.
The first interesting thing to note is that the best prediction threshold remains basically the same with the value giving the best improvement in performance and accuracy being 0.
In line with our last analysis the posterior probability increases till around 0. The maximum posterior probability is also much higher with a value of around This value represents the probability that a system gives a profitable out-of-sample result given that the system is classified positively by the machine learning algorithm.
Forex Mechanical System: SMA Crossover Pullback - gohabizaw.web.fc2.com
In line with normal classifier behavior the specificity increases as a function of the classification threshold while the sensitivity decreases, however at the optimum classifier threshold of 0. A higher sensitivity means that an OOS profitable system now has a higher chance of testing positive in the random forest test, implying that our performance now comes from a portfolio of trading systems that is larger than before because we simply trade more systems.
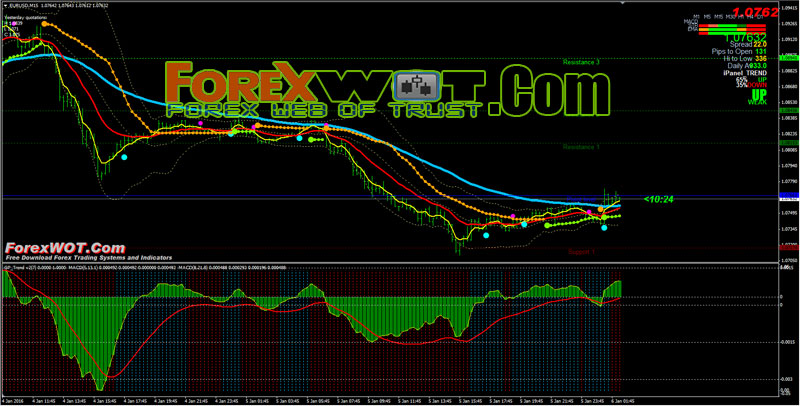
Overall the additional data we have gathered seems to point to the fact that our classifier has improved substantially from a Bayesian perspective. Not only do we have a higher sensitivity with a higher posterior probability but our improvement in performance has also increased dramatically compared with our last analysis.
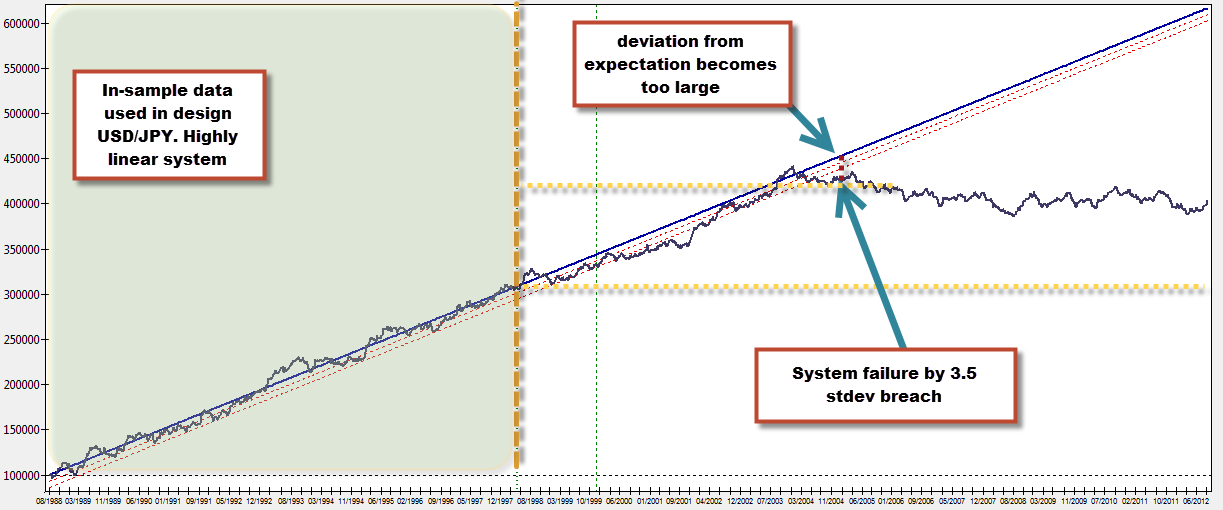
This is very encouraging as it means that additional data is providing our model with information that is useful from a forward looking perspective. Although we now have more than 5K points these still do not represent the entirety of systems we have mined — since we have lots of systems that do not have the necessary six months of out-of-sample performance to be included yet — so we should be able to double the size of this set during the next six months as systems we have already mined accumulate out-of-sample data.
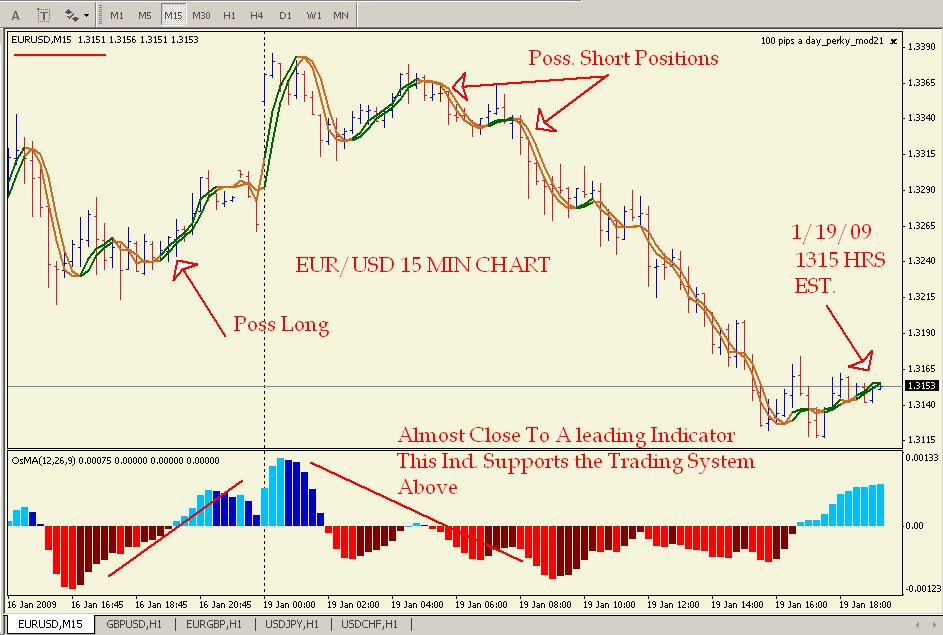
Once we achieve this I will repeat this analysis so that we can see if the model continues to improve or reaches a point of diminishing returns. Mechanical Forex Trading in the FX market using mechanical trading strategies. Home About Me Atinalla FE OpenKantu System Generator.
Is our random forest model for OOS predictions improving with time? June 11th, No Comments. Posted in Articles Tags: Asirikuy Asirikuy Investment Project Asirikuy Strategy Tester AST backtesting BATS brokers CFDs cloud mining crossword puzzles Currency Trader Magazine F4 framework fxtradermagazine grid trading homework corner indicator series Kantu machine learning martingale Metatrader metatrader 5 money management neural networks ODROID-XU4 openKantu pkantu portfolios portfolio trading programming psychology pyfolio python qqpat quantopian R RTFF scalping Seasonality social trading strategy design strategy evaluation system system development trading psychology trading strategies tutorial umaki Using R VPS walk forward analysis Watukushay WRP contributions.